Xiaohui Yu
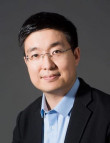
Professor
Graduate Program Director
Office: 3050 Victor Phillip Dahdaleh Building (DB)
(Formerly known as Technology Enhanced
Learning Building)
Phone: 416-736-2100 Ext: 33887
Email: xhyu@yorku.ca
Primary website: Personal Website
Secondary website: Google Scholar Profile
Media Requests Welcome
Accepting New Graduate Students
I am a Professor and the Graduate Program Director in the School of Information Technology, York University. I received my BSc degree from Nanjing University, China, MPhil from the Chinese University of Hong Kong, and PhD from the University of Toronto. I am affiliated with the graduate programs in Information Systems & Technology, Electrical Engineering & Computer Science, and Mathematics & Statistics at York University.
My current research interests lie primarily at the intersection of data management and machine learning (ML), including designing next-generation ML-based database components for more efficient query processing, as well as developing new algorithms and systems for large-scale ML. I am also interested in managing and analyzing spatio-temporal data arising from a variety of contexts, such as intelligent transporation systems, location-based services, and social networks. I am grateful for the generous support from NSERC, BRAIN Alliance, and leading industry partners. Our research results have been published in top database and data mining venues, such as SIGMOD, VLDB, ICDE, and TKDE.
Degrees
PhD, University of TorontoMPhil, Chinese University of Hong Kong
BSc, Nanjing University, China
Research Interests
- Dean's Award for Excellence in Research - 2009
- ACM CIKM Service Award - 2014
Current Research Projects
-
Summary:
- Dean's Award for Excellence in Research - 2009
- ACM CIKM Service Award - 2014
Fueled by the prevalence of capturing devices such as smartphones and cameras, we have witnessed an explosion of video data over recent decades. According to Cisco, video is expected to make up 82% of Internet traffic by 2022, with approximately 120TB of video data crossing the Internet per second. Such videos contain a wealth of information to be tapped to benefit our productivity, safety, and quality of life. However, they are heavily under-utilized due to the limitations posed by our current way of querying and analyzing videos.
On the other hand, the vast advances in Deep Learning (DL) in recent years have revolutionized numerous applications of major practical significance, including computer vision tasks such as object detection and object tracking. Integrating real-world applications with DL algorithms and models has become possible; understanding object types (e.g., person, car) and their locations in video frames is within reach by the application of specialized DL models. However, most existing video analytics solutions are purposebuilt and target specific applications; assembling the technical expertise to build and maintain the required
infrastructure and platform for video analytics that works across applications is still highly challenging, both in research and practice.
The proposed research program aims to address these challenges by developing solutions that bridge the gap between the need for powerful video analytics and the capability of specific DL models. The longterm objective of the research program is to develop the frameworks, models, algorithms, and systems for general-purpose structured video processing and analytics to unleash (through query processing) the vast potential of video data. As spatiotemporal information contained in videos is key to the processing of queries, the short-term objective is to develop spatiotemporal data structures, algorithms, and models for the processing of structured queries over video data. Advances will be made to address the following research questions: (1) how to retrieve clips from large video repositories with constraints on when and how objects appear; (2) how to quantitatively represent and store the evolving spatial relationships among a group of objects and perform efficient and accurate query processing over such relationships; and (3) how to re-identify the same objects across video clips based on their spatiotemporal association and visual similarities to support cross-video query processing.
The proposed research will help lay the foundation towards building a general-purpose video analytics system that is highly scalable and capable of performing fine-grained spatiotemporal pattern matching. It will help organizations and individual users uncover valuable information from videos and enable numerous applications in a wide variety of domains, such as video content creation, law enforcement, retail traffic analysis, and autonomous driving.
Start Date:
- Month: May Year: 2022
End Date:
- Month: Apr Year: 2027
Funders:
NSERC
Minor Research Grant (York University)
ATK Fellowship (York University)
Minor Research Grant (York University)
-
Summary:
The wide-spread use of smart phones, sensors and other IoT devices in cities world-wide has given rise to a huge volume of urban spatio-temporal data, which often present themselves as high-velocity continuous streams with considerable noise and uncertainties. These data record a vast amount of movement information of people, vehicles, etc., and serve as the backbone of a variety of applications, such as urban traffic management, road network planning, location-based services, and environmental monitoring. While governments, businesses and other organizations have realized the tremendous value of urban spatio-temporal data, how to effectively tap into this potential is still an elusive goal.
The unifying theme of the project is to address the challenges arising from managing and mining urban spatio-temporal data. Some of the questions we strive to answer are: How to improve the quality of such data to provide a reliable basis for data analytics? How to efficiently process continuous queries (such as k nearest-neighbor queries) and discover patterns over spatio-temporal streams? How to construct a probabilistic model to capture the underlying intention of movement? How to use this model to support advanced applications, such as traffic flow forecasting, dynamic navigation, and next location prediction?
Novel models and methods developed from this project will help lay the data management and analytics foundation for a wide spectrum of applications, and provide a better understanding of human mobility patterns.
Upcoming Courses
Term | Course Number | Section | Title | Type |
---|---|---|---|---|
Fall 2024 | AP/ITEC2610 3.0 | A | Object-Oriented Programming | BLEN |
I am a Professor and the Graduate Program Director in the School of Information Technology, York University. I received my BSc degree from Nanjing University, China, MPhil from the Chinese University of Hong Kong, and PhD from the University of Toronto. I am affiliated with the graduate programs in Information Systems & Technology, Electrical Engineering & Computer Science, and Mathematics & Statistics at York University.
My current research interests lie primarily at the intersection of data management and machine learning (ML), including designing next-generation ML-based database components for more efficient query processing, as well as developing new algorithms and systems for large-scale ML. I am also interested in managing and analyzing spatio-temporal data arising from a variety of contexts, such as intelligent transporation systems, location-based services, and social networks. I am grateful for the generous support from NSERC, BRAIN Alliance, and leading industry partners. Our research results have been published in top database and data mining venues, such as SIGMOD, VLDB, ICDE, and TKDE.
Degrees
PhD, University of TorontoMPhil, Chinese University of Hong Kong
BSc, Nanjing University, China
Research Interests
Awards
Current Research Projects
-
Summary:
Fueled by the prevalence of capturing devices such as smartphones and cameras, we have witnessed an explosion of video data over recent decades. According to Cisco, video is expected to make up 82% of Internet traffic by 2022, with approximately 120TB of video data crossing the Internet per second. Such videos contain a wealth of information to be tapped to benefit our productivity, safety, and quality of life. However, they are heavily under-utilized due to the limitations posed by our current way of querying and analyzing videos.
On the other hand, the vast advances in Deep Learning (DL) in recent years have revolutionized numerous applications of major practical significance, including computer vision tasks such as object detection and object tracking. Integrating real-world applications with DL algorithms and models has become possible; understanding object types (e.g., person, car) and their locations in video frames is within reach by the application of specialized DL models. However, most existing video analytics solutions are purposebuilt and target specific applications; assembling the technical expertise to build and maintain the required
infrastructure and platform for video analytics that works across applications is still highly challenging, both in research and practice.
The proposed research program aims to address these challenges by developing solutions that bridge the gap between the need for powerful video analytics and the capability of specific DL models. The longterm objective of the research program is to develop the frameworks, models, algorithms, and systems for general-purpose structured video processing and analytics to unleash (through query processing) the vast potential of video data. As spatiotemporal information contained in videos is key to the processing of queries, the short-term objective is to develop spatiotemporal data structures, algorithms, and models for the processing of structured queries over video data. Advances will be made to address the following research questions: (1) how to retrieve clips from large video repositories with constraints on when and how objects appear; (2) how to quantitatively represent and store the evolving spatial relationships among a group of objects and perform efficient and accurate query processing over such relationships; and (3) how to re-identify the same objects across video clips based on their spatiotemporal association and visual similarities to support cross-video query processing.
The proposed research will help lay the foundation towards building a general-purpose video analytics system that is highly scalable and capable of performing fine-grained spatiotemporal pattern matching. It will help organizations and individual users uncover valuable information from videos and enable numerous applications in a wide variety of domains, such as video content creation, law enforcement, retail traffic analysis, and autonomous driving.
Project Type: FundedRole: PI
Start Date:
- Month: May Year: 2022
End Date:
- Month: Apr Year: 2027
Funders:
NSERC
-
Project Type:
Funded
Funders:
Minor Research Grant (York University)
-
Project Type:
Funded
Funders:
ATK Fellowship (York University)
-
Project Type:
Funded
Funders:
Minor Research Grant (York University)
-
Summary:
The wide-spread use of smart phones, sensors and other IoT devices in cities world-wide has given rise to a huge volume of urban spatio-temporal data, which often present themselves as high-velocity continuous streams with considerable noise and uncertainties. These data record a vast amount of movement information of people, vehicles, etc., and serve as the backbone of a variety of applications, such as urban traffic management, road network planning, location-based services, and environmental monitoring. While governments, businesses and other organizations have realized the tremendous value of urban spatio-temporal data, how to effectively tap into this potential is still an elusive goal.
The unifying theme of the project is to address the challenges arising from managing and mining urban spatio-temporal data. Some of the questions we strive to answer are: How to improve the quality of such data to provide a reliable basis for data analytics? How to efficiently process continuous queries (such as k nearest-neighbor queries) and discover patterns over spatio-temporal streams? How to construct a probabilistic model to capture the underlying intention of movement? How to use this model to support advanced applications, such as traffic flow forecasting, dynamic navigation, and next location prediction?
Novel models and methods developed from this project will help lay the data management and analytics foundation for a wide spectrum of applications, and provide a better understanding of human mobility patterns.
Project Type: FundedRole: Principal Investigator
Upcoming Courses
Term | Course Number | Section | Title | Type |
---|---|---|---|---|
Fall 2024 | AP/ITEC2610 3.0 | A | Object-Oriented Programming | BLEN |